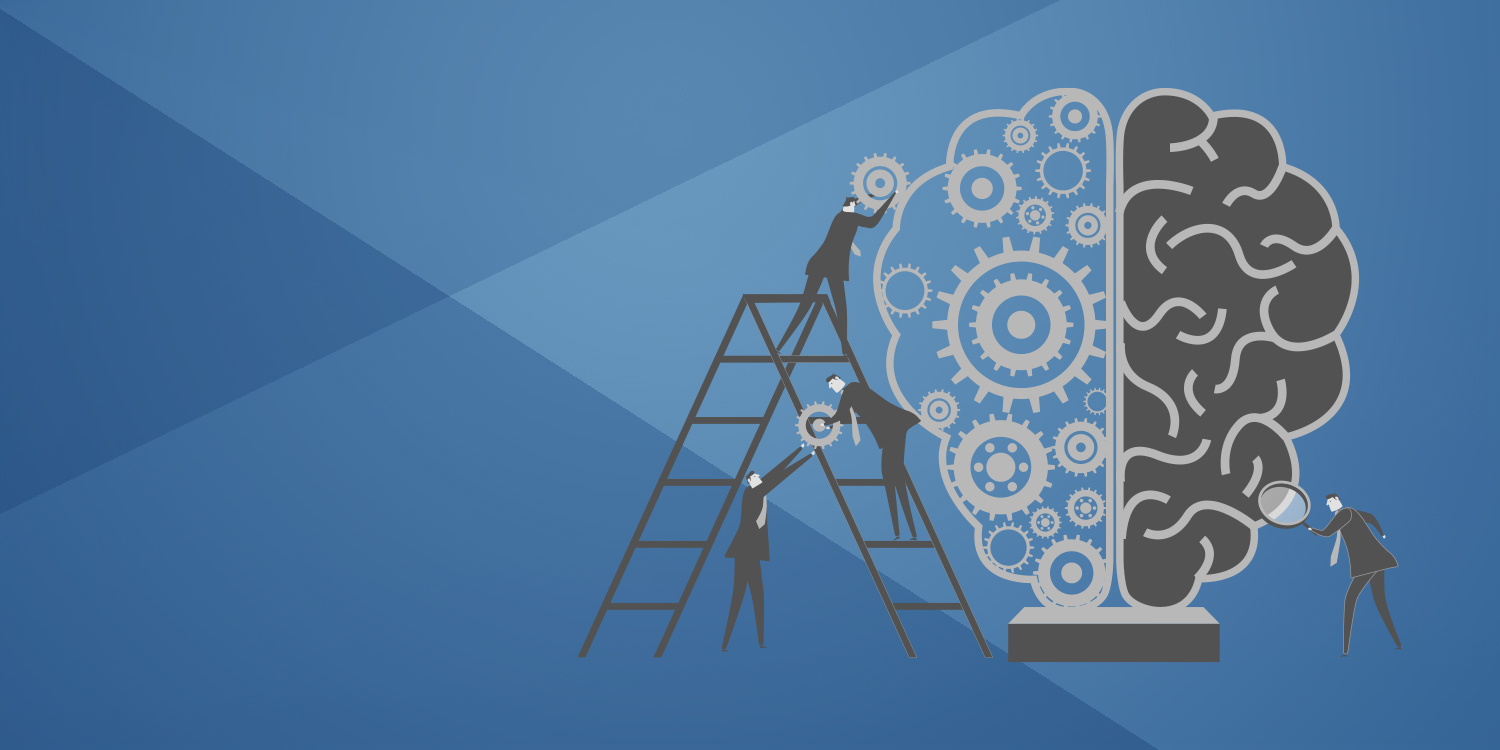
Artificial intelligence (AI) is a hot topic for medical research, and its potential for use in clinical settings is evolving rapidly—from improving diagnostic capabilities to gauging the likelihood of a drug’s effectiveness for a specific patient.
It's important for health care leaders to understand the fundamentals of the machine learning that is driving AI. This includes the steps involved in developing and validating new models, and how to harness its potential while avoiding potential pitfalls in health care applications.
What is Machine Learning in Medicine?
- Machine learning is a statistical approach to reasoning.
- There are three stages to developing a machine learning model: training, validation, and deployment.
- There are a variety of possible pitfalls at every stage of the process, and researchers should know what those hazards are to avoid them.
To dive into these points, we turned to Andrew Beam, PhD, an assistant professor at the Harvard T.H. Chan School of Public Health and at Harvard Medical School. Dr. Beam leads HMS Corporate Learning executive programs on the present and future possibilities for AI in medicine.
Machine learning involves a statistical approach to reasoning.
Machine learning comprises a series of algorithms to analyze data, learn from it, and make informed selections based on statistics.
Deep neural networks, also known as deep learning, are a subset of machine learning made up of many layers of artificial neurons. When researchers train the models, they create, connect, and reinforce artificial neurons to create an artificial synaptic connection pattern.
“The data makes some of those connections stronger, and makes others weaker,” says Dr. Beam. “We’re starting with this random artificial brain, and we’re reinforcing some of those artificial synaptic connections and pruning other ones using data. What we're left with is an artificial neural network that can do the thing that we want it to do.”
The three stages to developing a supervised machine learning model.
Stage one is training the model: Researchers feed the system with labeled examples of what they want it to learn. Training requires substantial amounts of historical data in a suitable format for the models. Not all data are created equal — for example, images are easier for the system to learn from than spreadsheet data.
Stage two is validation: Researchers take the model from stage one into a realistic health care setting. There, they validate that the model does what is intended by checking the model’s findings against the true outputs provided by human annotators. Researchers ensure that the performance characteristics are reflective of what was seen in stage one.
The human annotations that ensure researchers are training the models accurately are called labels. They are often expensive and time-consuming to collect but are usually the most important part of building a supervised AI system. Generally, a doctor, nurse, or other health care professional conducts a chart review or looks at an image to provide a label, which could be a diagnosis or a prognosis. Ideally, multiple people annotate every piece of data to ensure high-quality labels.
Stage three is deployment: The model is put to use in a research or clinical setting.
Understand the range of possible pitfalls in the process.
The model can fail if it doesn’t have enough data or if it’s hard to integrate into a real clinical workflow. Another pitfall is that the deep learning model can be trained to detect the wrong thing.
For example, consider a model intended to diagnose diabetic retinopathy and trained on retina images of patients, including those whose retinopathy has been treated. Rather than learning to recognize the disease, the model learns to recognize scars from earlier treatments, rendering the model useless for improving initial diagnosis.
Once a model is implemented in clinical practice, there are still challenges. If the hospital switches electronic health records (EHR) systems and the format of data changes, a machine learning model relying on the old system will need to be updated.
The bottom line? Always look for hints that the model is missing the target or may be hard to incorporate into clinical practice.
Before starting a machine learning project in medical research, it's essential to lay a strong foundation. Consider these three critical questions as a guide:
- Will it be able to predict or automate a task that improves your capabilities?
- Do you have access to large amounts of historical data?
- Is it the “right” kind of data?
AI in medical research is an exciting, burgeoning field, but it isn’t always the best use of resources. “AI does not free you from good statistical practice,” says Dr. Beam. “In some sense, it makes things like study design even more important, because it's very easy to mislead yourself. Just because you’re using machine learning and artificial intelligence doesn’t mean that you get to forget about biostatistics.” Some familiar methods, like statistical analyses, are easier and cheaper to conduct and work with text and spreadsheet data. For example, researchers have found that machine learning works better on diagnostic medical imaging tasks than predictive tasks.
“The pipeline from idea to clinical deployment is very long and complicated,” says Dr. Beam, “but I’m super excited.”
--
The Designing and Implementing AI Solutions for Health Care executive program from HMS Corporate Learning equips participants with a comprehensive understanding of key principles, technical nuances, and potential challenges in deep learning and emerging AI methods. Through a combination of live virtual class sessions, small group application exercises, pre-work, and robust discussions, participants will learn to evaluate organizational requirements and capabilities for AI adoption across diverse contexts, from large enterprises to startups.
Andrew Beam, PhD, is an assistant professor at the Harvard T.H. Chan School of Public Health and at Harvard Medical School. He is also the head of machine learning at Generate Biosciences, Inc., and leads research that uses machine learning to garner clinical insights. He received a Pioneer Award from the Robert Wood Johnson Foundation for his work on medical artificial intelligence.
Corporate Learning Blog
View the latest stories from our community members and expert partners at the Harvard Medical School Corporate Learning blog.