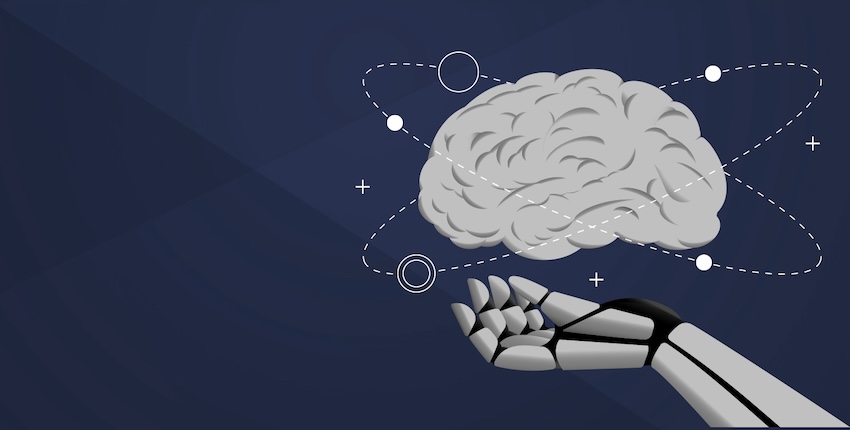
Imagine opening a massive spreadsheet of course feedback. The quantitative data - those neat columns of Likert scale responses - are easily graphed and analyzed. But then you scroll to the comments section, a sea of text full of valuable insights, yet more challenging to work with in a principled way. While we've mastered the art of crunching numbers, the richness of qualitative feedback, whether it be from classroom courses or corporate training, often eludes systematic analysis, relegated to ad hoc reviews that may miss crucial patterns. Our recent research explores ways to change this, making the analysis of text-based feedback as accessible and rigorous as its numerical counterpart.
In our recent study published in the International Journal of Artificial Intelligence in Education, my colleagues and I investigated an approach to this challenge: the use of large language models (LLMs) such as GPT-4. These advanced AI tools have demonstrated remarkable capabilities in understanding and processing human language, opening new possibilities for analyzing qualitative data, such as the feedback that learning and development (L&D) leaders use to help guide their decisions.
Our research showed that LLMs can effectively perform a range of complex tasks crucial for survey analysis, including categorizing comments, extracting relevant information, identifying themes, and assessing sentiment. Notably, GPT-4 achieved human-level performance on these tasks without requiring specialized training data. This suggests the potential to analyze thousands of comments in minutes rather than days or weeks.
As an example use case, imagine a common scenario faced by many L&D professionals: evaluating the effectiveness of specific training components within a larger program. Let's say you run a training program for new employees in a pharmaceutical company's drug development division. Two years ago, you introduced a drug discovery simulation exercise as part of the onboarding experience. While valuable, the simulation requires significant resources to maintain, and your team is considering replacing it with a new module. You have collected over 500 responses to open-ended feedback questions on your standard post-training survey. However, the survey doesn't have a dedicated question about the simulation, making it difficult to gauge its impact solely through manual analysis. How can you make a data-driven decision about the simulation's future?
Traditionally, L&D teams might skim through a subset of comments, potentially focusing on those that are longer or emotionally charged, without a clear understanding of overall learner sentiment. This approach is time-consuming, prone to bias, and may lead to incomplete insights. However, new AI tools built with LLMs offer a powerful alternative. Imagine being able to quickly and easily: 1) identify all comments mentioning the simulation; 2) extract only the relevant portions from those comments; 3) analyze the sentiment expressed towards the simulation across all extracted portions; and 4) present the results in a clear, quantitative way, including sentiment scores and the number of relevant comments, alongside the actual text. Having this type of analysis readily available (fast enough to potentially even be run during a team meeting) empowers L&D professionals to make balanced, data-informed decisions about program content and resource allocation.
The implications for education, including professional training, are significant. By making high-quality qualitative analysis more accessible and efficient, LLMs could enable educators to quickly identify areas for program improvement, respond more rapidly to participant needs, and make data-driven decisions with greater confidence. This technology has the potential to uncover insights that might be missed in manual review, enhancing the overall quality of education.
While our study focused on education, the applications of this technology extend far beyond. Any field dealing with large volumes of text data – from market research to customer feedback analysis – could benefit from these AI-powered techniques. As AI continues to evolve, we may see a shift in how organizations across industries derive insights from unstructured data, adding a powerful tool in decision-making processes.
Michael Parker, MD, is the associate dean for online learning research, faculty director of HMX, and an assistant professor of medicine at Harvard Medical School. Read the full study here.
Corporate Learning Blog
View the latest stories from our community members and expert partners at the Harvard Medical School Corporate Learning blog.